Data is in The Spotlight for Financial Services
The financial services industry — from the boardroom to the customer — finds itself in a transformative era. Banks, insurers, and financial services firms are hyper focused on expanding existing relationships and attracting new customers. They’re doing it by putting an operational spotlight on cultivating more immersive experiences while simultaneously curbing costs — with an eye on AI as the magic bullet to achieve it all. It’s being driven by the need to meet elevated customer expectations for personalized services and products. It’s clear customers will go to whatever organization provides them the best experience, and the competition is also racing to adopt AI as the barriers to entry are low
62% of customers said they would switch financial services providers if they felt they were being treated as a number, not a person.
73% of customers expect companies to understand their unique needs and expectations.
But in the mad dash to align operational transformation with evolving customer expectations, many analytics and IT leaders are ignoring the basics: The potential of AI to solve these problems is intertwined with the quality and trustworthiness of the underlying data. There must be an understanding that the diligent, yet sometimes time-consuming work, to ensure data is accurate and complete is a necessary investment for the payoff of high-quality insights. The basic, simple work to create a data foundation at the outset is necessary for any future AI for financial services initiative. It’s clear industry leaders recognize this vital connection.
87% of Financial Services Analytics and it leaders say advances in AI make data management a high priority.
93% of financial services business leaders feel their organizations should be getting more value out of its data.
Many financial services institutions have at least recognized the need for a comprehensive data strategy and integration platform to empower their teams with the insights they need to reap the benefits of AI and win in the marketplace. Organizations have two mountains to climb: tapping into all of their data that sit in silos disconnected from legacy systems and advancing their use of data — call it data maturity — while also staying compliant with data privacy and regulatory rules. The good news is that the strategy is straightforward and doesn’t require huge investments in building out a larger IT team. This data maturity playbook for financial services outlines the steps needed to advance the use of data in banking, insurance, and wealth and asset management to scale capabilities, deliver value, and empower the journey to AI maturity.
Working Definitions: Data Strategy, Data Integration, Data Maturity
Data Strategy
Simply put, a data strategy is the foundation for hitting company goals with the use of data analytics. But it’s not one size fits all. First, you need to make sure your business objectives are clear and employees have been trained in data accuracy and security. Why does this matter? Data is the key to making savvy business decisions, streamlining internal processes, growing the business by targeting new and existing customers, and personalizing their journey with your brand. A data strategy, or a path to data maturity, is the key to helping your company identify where customers or prospective customers are in their journey and guide them in their decision-making process.
Data Integration
Data integration is the group of technical and business processes happening behind the scenes, bringing together data that is scattered across various divisions of your organization, or with outside vendors, into one source of meaningful information. For example, data can live in legacy, SaaS, custom, and/or core systems, and it can also come in various formats such as structured or unstructured. Data integration is automated and streamlines the process of requesting and combining all that data — no matter the source — into a unified, single source of truth that can be accessed by anyone or any system within the organization.
This process also includes identifying where the data needs to go after it’s been harmonized – that is, if it needs to flow to another system as part of a process or automation.
Data Maturity
A data mature organization has transformed itself by using data to make key day-to-day decisions, drive innovation, and plan for the future. Those rewards are clear: Data-driven companies make better decisions and accomplish goals faster. It goes beyond a pure digitally driven transformation, as well; data mature organizations have also evolved their culture — meaning every person is equipped with the insights and training needed to be data-driven.
Data and analytics are simply part of every aspect of operations: aligned to business outcomes, prioritized in decision-making and processes, and united in a shared mission to lead with data. Data maturity is also the foundation for AI success. Data mature organizations are twice as likely to have the high-quality data needed to use AI effectively.
SMBs face unique challenges
According to a report from Apiture and Aite-Novarica Group, 60% of decision-makers indicated they would likely switch to a smaller bank, like a community bank or credit union, if its products, services and customer experience were comparable to those of a larger bank. The larger money centers have larger talent pools and IT resources to spend on the latest technology. However, smaller to mid-sized business (SMBs) have to compete on service and local convenience. The world is rapidly changing as AI democratizes and scales relationship based knowledge and services. SMBs face unique challenges when it comes to data maturity — even though the core principles of strategy, implementation, and, ultimately, importance remain the same.
Here is what SMBs should consider when pursuing data maturity without the resources available to dedicate to data management or to invest in advanced data technology relative to larger enterprises:
Establish Clear Goals
It is critical to identify what aspects of the business you want to improve with data. Defining your goals will focus your efforts and prioritize the steps you take to achieve data maturity. You can then identify the types of data critical to achieving those goals. Starting with data critical to your goals can save time, capital, and human resources that would have gone into solidifying all of your organizational data.
Choose Technology Over Teams
Many SMBs lack the human resources or suffer from a skills gap required to build out an IT team to take on a data maturity project. Choosing scalable cloud technology solutions grows with organization rather than building out a costly IT infrastructure.
Ensure Culture is Aligned
Effective change management strategies and leadership support are essential to overcoming a resistance to the change to a data-driven culture, which can be common in SMBs. Equipping the team with the right skills to embrace data maturity is equally important to ensure complete buy-in to data-first decision-making.
Why do leaders in financial services need data maturity now?
Having quality, trusted information at the right time and in the right context is crucial for business success and has been for some time. Now, AI has upped the ante and increased demand for data maturity, a clear payoff from the data investment. In fact, Salesforce found that survey respondents in high-maturity organizations were twice as likely to have the high-quality data needed to use AI effectively. For IT and analytics leaders in financial services, top challenges around data include security threats, lack of data harmonization, too many manual processes, budget constraints, and limited workforce data skills.
91% of financial services analytics and IT leaders say the need for trustworthy data is higher than ever.
Effective data integration helps resolve these challenges as it allows teams to make smart decisions faster and with more accuracy than ever before, while eliminating blockers that impede collaboration. A comprehensive data strategy then empowers companies to harness that data and transform how they operate and deliver rich customer experiences. That’s only enhanced by AI. But without first getting your data in order and structured in a way that is easily accessible across departments — data maturity — an effective AI strategy becomes impossible. Leaders need to empower everyone to lead with data maturity and build a trusted data foundation across their organizations.
Five Steps to Data Maturity
1) Set goals and identify manual data processes
Data strategy is not one size fits all; it must be tailored to the specific needs and goals of your business. You’ll need to start by determining the goals and KPIs you are trying to achieve, identifying your data, assessing its current state, defining the best approach to make sense of your data, and identifying the right team and software to execute that strategy.
Determine your goals.
To align business and data priorities, you’ll first need a clear understanding of your organization’s goals — including those of key senior executives and leadership. Meeting with these stakeholders and getting a sense for their priorities and needs is the first step in developing a unified cross-functional view of your data. These goals can be overall optimization companywide or incremental, such as improving customer service, risk assessment, fraud detection, etc.
Identify your data and assess its current state.
With leaders on board and a unified view of your end goals, you can now examine your data to identify what you have and what you need. Identifying data sources across your institution and every customer touchpoint, how each source can be accessed and the quality hierarchy of the sources is a crucial early step. Then, manually pull data from those sources to populate reports. Once this is achieved, it may also shine a light on what additional data you may need to achieve your goals.
Identify your use case.
It’s important to identify a use case up front once you’ve unified your data. While this use case will be unique to your business, we’ve pulled together some common examples across banking, insurance, and wealth and asset management:
Salesforce Industry Use Case: Financial Advisor
A financial advisor’s goal is to provide tailored investment advice and financial planning services to clients, and identify new market opportunities and optimize asset allocation strategies. This includes evaluating client financial data, investment portfolios, market trends, and economic indicators. Financial analysts, data scientists, portfolio managers, and client relationship managers all use analytics tools for portfolio optimization, risk assessment, and predictive modeling. All of these data points are used to understand their financial goals, risk tolerance, and investment preferences. Unifying this data is the first step toward developing AI algorithms to track client interactions, preferences, and investment performance, enabling proactive client engagement and personalized service delivery.
Salesforce Industry Use Case: Insurance Carrier
An insurance carrier faces several organizational challenges managing an influx of first- and third-party data, all while optimizing policyholder satisfaction by offering personalized products and enhancing operational efficiency. Doing this requires accessing data from multiple sources (telematics, IoT, underwriting exchanges, public records, etc.), analyzing weather patterns, risk profiles, claims history, and rating trends. Conducting a data maturity audit allows the implementation of AI-powered analytics platforms for risk assessment, customer segmentation, and predictive modeling — resulting in unified customer profiles. Now, when a policyholder initiates a call or chat, the insurance carrier and their distribution partners are empowered to improve the customer experience immediately and recommend personalized products such as home and auto bundles or usage-based insurance.
Salesforce Industry Use Case: Retail Banking
Banking service agents often need to access a multitude of systems (deposit, loan, credit card, etc.), each of which has its own set of customer data. This requires that the agent ensure they are looking at the same customer in each system. For example, Jon Doe could also be identified in a different system as J. Doe. Ensuring they are the same person interferes with the customer interaction and slows down the issue resolution process. Once data has been integrated into a single, harmonized view of the customer, the agent can feel assured they have all the information needed about this customer in one place. This view gives the agent a complete picture of the customer’s financial relationship with the bank, recent transactions, interactions, with the ability of AI to surface up relevant recommendations or alerts in real time. Now, when the agent needs to resolve a common service request, like a transaction dispute, they’re empowered with the right data. This unified customer view is shared across the contact center, branch, and middle-and back-office teams, so when the agent collects the necessary information about the transaction in question, details are seamlessly passed to the fraud department to investigate. This results in faster case resolution, a better service experience, and increased customer satisfaction.
02) Reduce multiple, inconsistent sources of truth
Many organizations are plagued with legacy data that is spread throughout multiple systems in various spreadsheets, PDFs, emails and scanned documents created by different employees. The data has been input manually and is riddled with inconsistencies and/or errors. Employees are slowed down looking for what they need as they search for the right data from different sources, all to create a reporting presentation to send up to their manager, wasting time and resources. You’ll need to work with stakeholders across your organization to identify, locate, and integrate these key sources of information. This also underscores the importance of semantic search — an advanced search technique that factors in the user’s intent behind a query — which adds a layer of intelligence and context to the process of data integration. Semantic search enhances the process by interpreting data even as terminology and context changes over time.
Identify manual data processes from disparate sources.
Once all of the sources are identified, you can begin bringing the data together. This process allows you to analyze the data from different sources and strip away repetitive data points, discrepancies, and inconsistencies. It is also important to establish a data governance plan, the rules or policies by which companies collect, manage, and store information. This is crucial for winning customer and employee experiences with trust at the heart of every interaction. It’s important to include transparency in decision-making processes and implement fairness and bias mitigation (especially important in areas such as credit scoring) to build and maintain trust.
Leverage business intelligence tools.
You’ll also need to choose the best business intelligence software that gives you the visualization tools to leverage your data through a single source of truth. You should look for technology that can connect and harmonize data from any product and system into a complete view of every customer, using AI to empower your team to act on relevant data and insights from your existing processes and applications. Here you can also prioritize the standardization of data formats, definitions, and automate entry methods to establish a reliable dataset.
03 Tell the full story
With your data integrated, it begins to tell a story. But you’ll need to clarify what that narrative is. Here you can clearly define what questions you want answered now that you possess a complete view of the data. Data integration creates a single source of truth and brings business objectives and IT together. This gives your teams all the tools necessary to achieve their goals. This can only be successful when security is a priority, especially when integrating sensitive customer data. Any breach, large or small, will destroy customer trust and deteriorate many of your larger strategy goals. The protection of your customer’s data is paramount.
Take a simplified, role-focused approach.
Focusing on specific employee roles allows you to answer the most important questions (i.e., are we recommending the best financial instruments to clients, is our customer service optimized, do our analysts have a complete view of the customer). To prevent inefficiency, however, the aggregated data must be stripped down to what is required to effectively answer questions for each specific role. In other words, you want to prevent death by dashboards, or a data overload where employees click from graph to graph to find the answers they need. Answering role-specific questions allows you to give them the answers they need on a single screen.
Develop guidelines for sharing data with customers and vendors.
As your data strategy matures, valuable insights will begin to emerge. Naturally, employees will want to share those insights with customers to show increasing value. This is where your data governance plan comes in. Staying on top of data security — i.e., a customer’s personal identifiable information or critical proprietary data — is critical to prevent privacy breaches, while also adhering to any relevant industry standards and regulations.
Revisit your data governance plan.
Data governance refers to the rules or policies by which companies collect, manage, and store information. The governance of your data matters because it’s core to how companies deliver winning customer and employee experiences with trust at the heart of every interaction. Staying on top of data governance for key data is critical to reduce duplication errors and privacy breaches, while also adhering to any relevant industry standards and regulations. It’s important to include transparency in decision-making processes and implement fairness and bias mitigation (especially important in areas such as credit scoring) to build and maintain trust.
Secure data infrastructure and processes.
It is imperative to follow security best practices and use available tools to strengthen the security of your data. At Salesforce, this means implementing technical security measures such as protections against system vulnerabilities, logical separation of customer data, robust network security, encryption of data in transmission, and options for encryption of data at rest. Also, it is critical in the financial services industry to deploy cybersecurity solutions to detect threats such as data breaches and formulate response plans.
Ensure due diligence and ongoing review.
When conducting due diligence, you should gain a clear understanding of your chosen data management platform and its underlying architecture. Then, using that technology’s framework, tools, and measures, meet and demonstrate compliance with applicable rules and regulations via ongoing review.
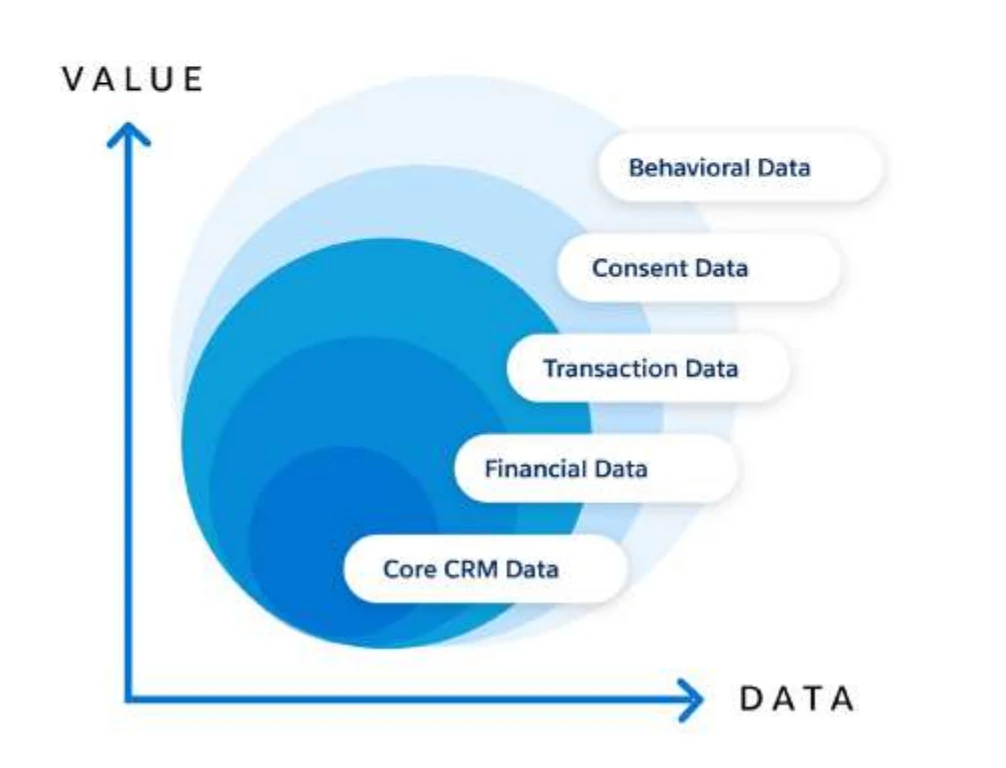
04 Get intelligent insights
Your goals have been set, key stakeholders are on board, your team, data, and platform are ready to go, and you have secure data infrastructure and processes in place. Now, you need to unlock your strategy with your unified customer data, AI, and automation. A combination of CRM + AI + Data + Trust means your data is working to tell a story of your customers and their needs — creating customizable, predictive, and generative AI experiences to fit all your business needs safely.
Get smarter relationship insights, faster.
Make every customer interaction productive when you empower your front office to discover and surface key insights about their customers and their financial needs. This includes predictive AI and algorithms to forecast trends, customer behavior, and market dynamics. Reduce the cost to serve with automation with self-service options such as AI-driven website journeys or chatbots. AI-driven insights can help customer service agents, armed with a complete view of the customer, provide proactive solutions to problems or guide customers to relevant insight.
Reduce your IT footprint.
With your organization’s data infrastructure in place, you can now implement an out-of-the box CRM that has built-in AI and trust components designed specifically for the financial services industry. This reduces development costs with prebuilt automations, built-in regulatory compliance, integrations with your core platforms and data.
Take personalization to the next level.
You can improve customer loyalty and retention with personalized experiences powered by real time financial data. Personalized Financial Engagement enables financial institutions to connect disparate data systems by creating a unified profile of each customer and delivering more automated and intelligent customer journeys at scale with generative AI.
Act on next-best-action recommendations.
This level of data maturity directly influences the sophistication and effectiveness of next-best-action recommendations. As you progress along your data maturity journey, it will continue to unlock the potential for more accurate predictions, personalized interactions, and ultimately a higher and higher level of customer satisfaction. The integration of advanced analytics with real-time processing and continuous data improvement snowballs, resulting in always improving next-best-action recommendations. This takes your customer engagement to a whole new level.
05 Transform your organization.
Achieving true data maturity is a goal organizations are constantly striving for. At this stage, your machine learning is humming, your data is clean and integrated, and your teams have the necessary skills. You’ve automated manual, repetitive tasks so your teams can focus on high level activities, using algorithms for insight-driven decision-making and AI-driven forecasting and next-best-action recommendations to improve client satisfaction. Meaning, your organization is truly transformed into a data-driven machine. There are, however, a few more steps you can take to truly optimize your business.
Proactive and reactive behavior.
With AI built into your organization, you can now ensure that actions being taken are proactive, in addition to reactive. For example, AI can flag to your team that a prospective client is browsing your webpage, down to the specific products they’ve checked out. These are insights that wouldn’t be available to your company without data maturity having set the foundation for AI. Now, when a salesperson contacts that prospect, they’re armed with information from those interactions they wouldn’t normally have. But it can go a step further; your built-in AI can deliver proactive recommendations on how to engage the prospect to ensure a successful interaction with your salesperson.
Empower employees to take on new challenges.
This type of digital transformation can cause trepidation among your employees — concern that this powerful technology will take their jobs. This is where empowerment comes in, to challenge them to take on new skills and embrace this technology to transition from low-value work to missions that add real value and innovation to your organization.
Industry Use Case: An AI Powered Financial Advisor.
An AI-powered advisor can analyze client financial data, investment preferences, and risk tolerance, enabling personalized investment strategies, asset allocation recommendations, and financial planning services. This could be something as simple as saving for a trip or something bigger like buying a home. AI can help identify a client’s needs based on their profile and investment history and help make investment recommendations based on their time horizon. Typically, the more time you have, the more risk tolerance there is. Integrated AI capabilities can also help manage client relationships and track investment performance, enhancing client engagement and satisfaction.
Industry Use Case: Contact Center Banking Agent
AI-powered insights empower agents with the real-time information they need to turn service interactions into opportunities to anticipate and proactively serve customer needs. Let’s say a customer has called their bank to report a lost credit card and order a new one. Historically, the contact center agent would begin the service process to terminate the lost card, issue a new one, and then end the conversation once it was ordered. But now the conversation transforms into a proactive opportunity to better serve the customer. For example, the agent may see an alert that this customer qualifies for a more financially beneficial credit card. The agent can now suggest an alternate product offering better suited to the customer’s needs. This type of service experience shows the customer that their bank is not just responsive but also deeply understands their needs and is offering ways to improve their financial wellbeing, which drives better satisfaction and loyalty.
Salesforce Industry Use Case: Insurance Broker
Insurance brokerages face challenges in aggregating and maintaining data from various sources. This segment must overcome persistent issues with data residing in many data repositories and agency management systems. To drive meaningful, organic growth and highlight their value to carriers and clients, insurance brokerages need to eliminate siloed or disparate legacy systems and create complete client profiles powered by real-time data. Conducting a data maturity audit allows these businesses to deliver AI-driven sales, service, and marketing functions. For example, insurance brokerages can — in conjunction with an AI platform — prioritize profitable sales opportunities, deliver personalized client service/coverage recommendations, mitigate attrition risk, and optimize carrier relationships.
Industry Use Case: An AI Powered Financial Advisor
An AI-powered advisor can analyze client financial data, investment preferences, and risk tolerance, enabling personalized investment strategies, asset allocation recommendations, and financial planning services. This could be something as simple as saving for a trip or something bigger like buying a home. AI can help identify a client’s needs based on their profile and investment history and help make investment recommendations based on their time horizon. Typically, the more time you have, the more risk tolerance there is. Integrated AI capabilities can also help manage client relationships and track investment performance, enhancing client engagement and satisfaction.
Customer Data Powers AI on Bank’s Digital Platform
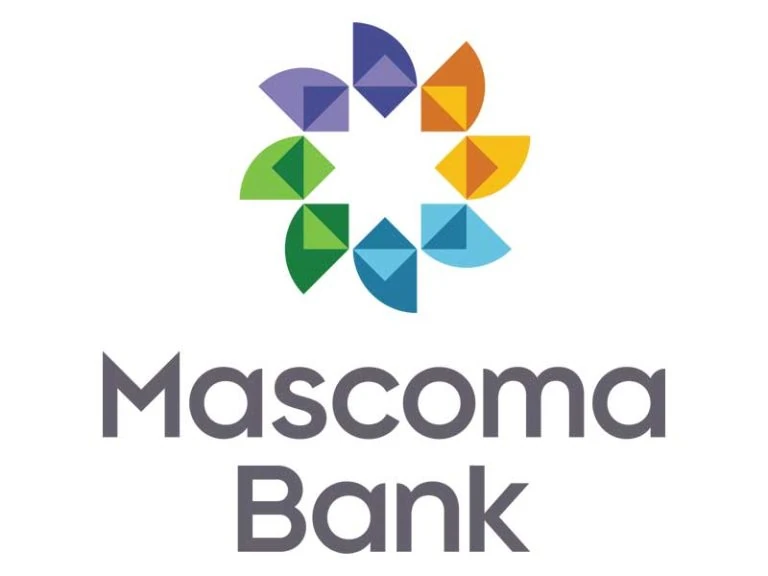
Mascoma Bank serves its communities and customers with a wide array of financial services including deposit products, loans, commercial banking, insurance, and wealth management. Mascoma was using time-consuming, disparate systems, email, and manual back-and-forth conversations, which was inefficient and hindered the ability to provide personalized services.
Mascoma now leverages Financial Services Cloud to automate numerous outdated, multistep processes, thereby enhancing its ability to achieve first-contact resolution for its customers. It will also apply its customers’ unified data to power valuable insights with AI. Financial Services Cloud provides the bank with one platform that all its employees can use to access a 360-degree of customers and their relationship to the bank. It gives agents at any branch all the relevant information for a successful interaction without the customer having to repeat their information to several agents. The platform also enables agents to provide proactive recommendations and cross-sell opportunities, a capability that will further advance with the integration of AI.
Mascoma Bank helps customers achieve financial wellness with data
- One unified system of record for all customer information
- Transformed manual ticketing systems for eight lines of business into automated ticketing queues • Consolidated data maintenance processes to gain efficiencies and improved CX
- The rapid identification of significant customer pain points using call center tagging
- The streamlining of 800 process user stories into 100 automated workflows
- The processing of one years’ worth of loans in 13 days
Deliver Personalized Experiences with CRM + AI
Achieving data maturity for successful AI implementation requires a strategic and systematic approach from start to finish, identifying company goals, ensuring governance and security, and finally, launching a generative CRM. The investment into that data foundation is what results in the payoff of AI-driven growth, innovation, and customer satisfaction.
The next step is making sure AI for financial services can answer questions accurately and expertly. Our vision is to solve for that accuracy through prebuilt AI actions and use Data Cloud semantic search to power the intelligence for those actions.
Data Cloud for Financial Services Cloud connects and integrates customer data, such as interactional, behavioral, and transactional data across systems to give financial institutions a unified profile of each customer and real-time insights. For example, a banker can be alerted if a customer has a large balance in a standard savings account. This allows the banker to proactively reach out to suggest transferring the balance to a more beneficial financial instrument like a high-yield savings account or CD.
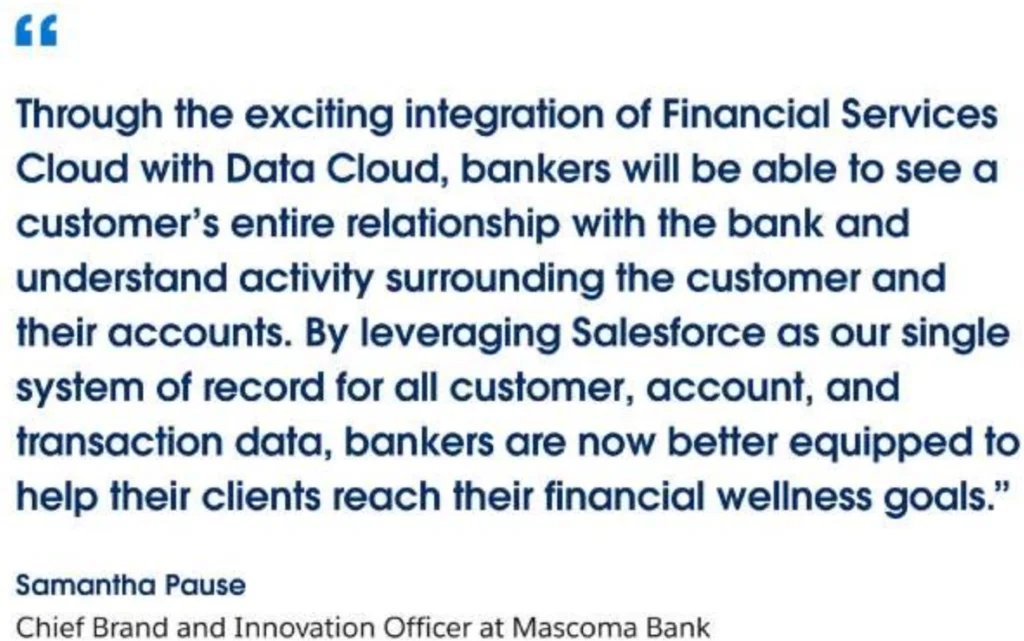
By using Salesforce’s built-in AI from Einstein to create insights and recommendations, combined with powerful automation from Flow, Data Cloud powers the first-ever real-time CRM.
With Financial Services Cloud, this empowers every firm to connect and harmonize their customer data. Data Cloud for financial services was purpose-built for the Salesforce Platform and transforms every experience inside Salesforce, giving your organization that single view of your customer across sales, service, marketing, commerce, and more. The built-in automation and AI allows you to improve efficiency and reduce risk by reusing business processes across channels, automating data collection, document generation, and workflows. It integrates with core banking and insurance platforms to connect financial, transaction and customer data — along with best practices for data privacy and security to ensure regulatory compliance — right out of the box. This allows your organization to get results faster, drive innovation, and reduce costs.